Understanding the Importance of Semantic Segmentation Annotation Tool in Software Development
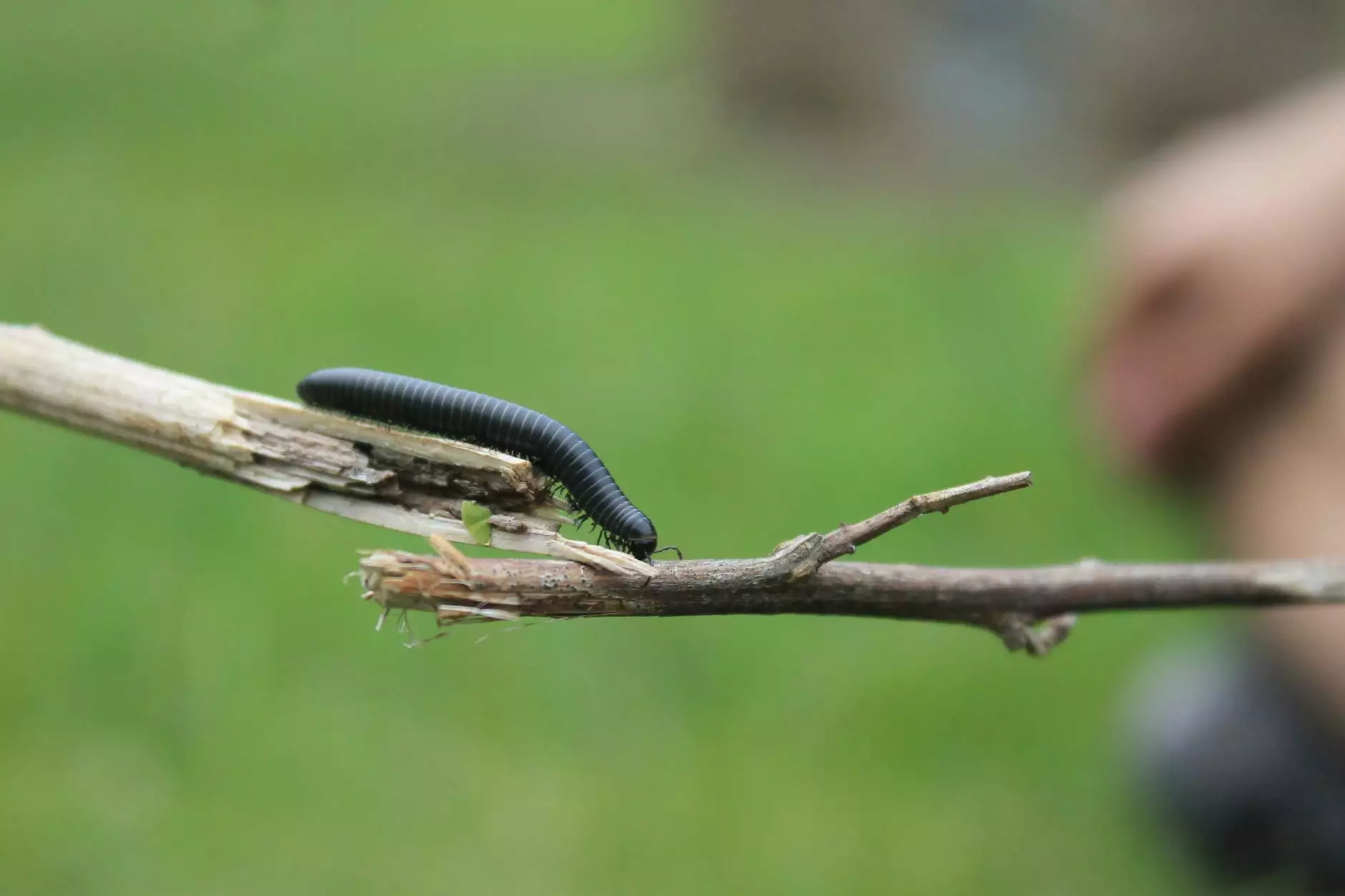
In the realm of software development, particularly in areas like artificial intelligence and machine learning, the utility of a semantic segmentation annotation tool cannot be overstated. As we delve into this article, we will examine what semantic segmentation entails, the role of annotation tools in its implementation, and the profound impact these tools can have on various industries.
What is Semantic Segmentation?
Semantic segmentation is a process in computer vision that involves classifying each pixel in an image into a category. Unlike traditional image classification—where the goal is to label an entire image—semantic segmentation provides detailed insight by pinpointing regions within images that belong to different classes. This allows for a more nuanced understanding of the visual context.
The Role of Annotation in Semantic Segmentation
At the heart of effective semantic segmentation is high-quality annotation. This is where a semantic segmentation annotation tool comes into play. These tools enable developers and data scientists to annotate images accurately so that machine learning models can learn to distinguish and classify objects within images effectively.
Benefits of Using Semantic Segmentation Annotation Tools
- Enhanced Image Recognition: Annotated data helps train models to achieve high levels of accuracy in object detection and classification.
- Efficient Training Data Creation: Automated tools speed up the process of generating labeled datasets, reducing the overall time spent on data preparation.
- Consistency and Precision: These tools often employ AI to maintain uniformity in annotation, ensuring that each image is categorized correctly.
- Scalability: As projects grow, these tools allow for the rapid scaling of datasets without compromising quality.
Key Features of High-Quality Semantic Segmentation Annotation Tools
Not all annotation tools are created equal. When selecting a semantic segmentation annotation tool, there are several key features to consider:
1. User-Friendly Interface
A tool with an intuitive interface allows users to annotate images efficiently. This reduces the learning curve and enhances productivity, enabling teams to focus on what truly matters—developing machine learning models.
2. Support for Multiple Formats
High-quality tools often support various image formats (JPEG, PNG, etc.), enabling flexibility in annotation. This is crucial as different projects may require diverse data inputs.
3. Collaboration Features
The ability for multiple users to collaborate on annotation projects in real time fosters teamwork and ensures that collective insights are integrated into the project's progress.
4. Machine Learning Integration
Advanced tools may offer built-in machine learning capabilities to assist in the annotation process, providing suggestions and automating repetitive tasks. This can drastically increase efficiency, allowing for a rapid turnaround on data generation.
Use Cases of Semantic Segmentation in Diverse Industries
The applications of semantic segmentation are vast, impacting various sectors:
1. Autonomous Vehicles
In the automotive industry, semantic segmentation is essential for developing self-driving cars. Annotating images from vehicle sensors allows models to be trained on road signs, pedestrians, lane markings, and more, ensuring safety and accuracy on the road.
2. Medical Imaging
Semantic segmentation plays a critical role in the analysis of medical images, such as MRIs and CT scans. By accurately annotating tissue types and lesions, healthcare professionals can improve diagnosis and treatment.
3. Agriculture
In agriculture, semantic segmentation tools help in analyzing crop health by classifying different plant types, detecting diseases, and optimizing yields through precise image analysis.
4. Augmented Reality
In augmented reality applications, semantic segmentation is crucial for accurately integrating digital objects with the real world. This enhances user experience and interaction in AR environments.
Choosing the Right Semantic Segmentation Annotation Tool
When you’re in the market for a semantic segmentation annotation tool, it’s important to assess your specific needs:
- Project Scale: Determine if the tool can handle the size of your dataset without compromising performance.
- Customization: Look for tools that allow for tailored annotation workflows to suit your projects best.
- Budget: Consider the cost-effectiveness of the tool vs. the features provided.
- Customer Support: Reliable customer support can greatly influence your overall experience with the tool.
The Future of Semantic Segmentation Annotation Tools
As technology continues to evolve, the future of semantic segmentation annotation tools looks promising. Innovations in artificial intelligence will lead to more advanced and automated annotation capabilities, reducing the manual effort required in the training of machine learning models.
Additionally, as industries continue to realize the potential of semantic segmentation, we can expect an increase in demand for more specialized tools tailored to specific sectors such as healthcare, automotive, and agriculture.
Conclusion
The importance of a semantic segmentation annotation tool in the landscape of software development cannot be overemphasized. These tools not only facilitate the creation of high-quality datasets but also drive innovation across numerous industries. As businesses increasingly rely on AI and machine learning, leveraging the right annotation tool will be vital for maintaining a competitive edge.
In summary, whether you are developing applications for autonomous vehicles, enhancing healthcare through medical imaging, or exploring agricultural innovations, the integration of semantic segmentation tools is critical to unlocking the full potential of your data.
Take the leap into the future of technology. Embrace the power of semantic segmentation annotation tools and transform your data capabilities today.